AI within the TOPdesk Platform: Putting our vision into action
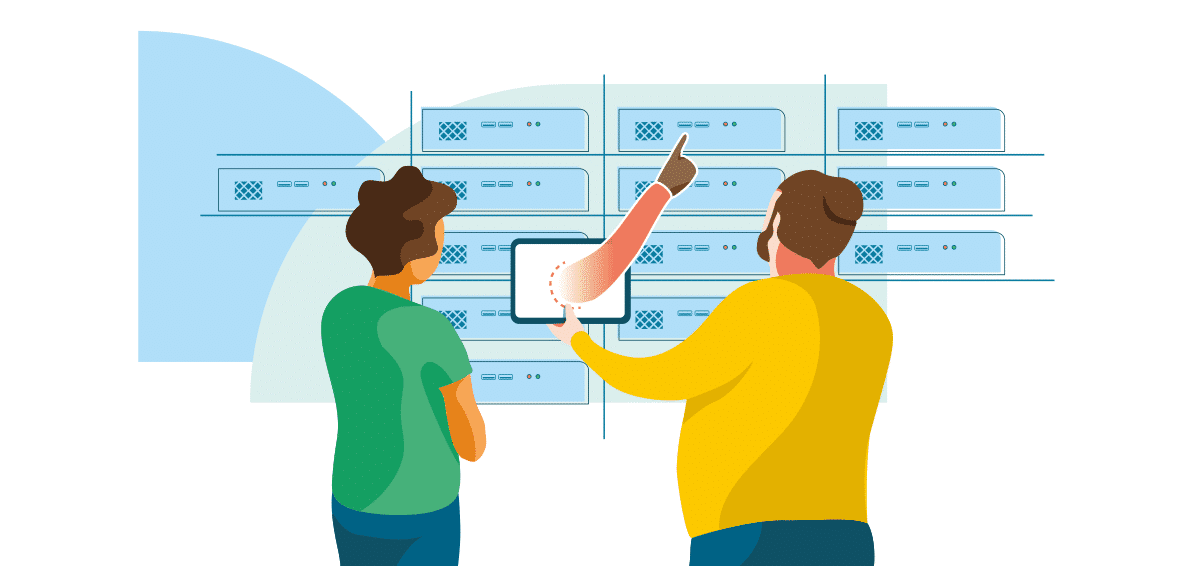
Back in June 2020, we laid out our overarching vision for using artificial intelligence (AI) both within the TOPdesk platform and within the TOPdesk Marketplace.
This blog is a follow-up to our initial vision, focusing primarily on how we are developing AI capabilities within the TOPdesk platform itself. We will soon also release a Marketplace-focused blog, but for the sake of clarity, we deemed it best to split these two areas into two distinct posts.
Throughout this post, l will outline the recent steps that we have taken with regards to leveraging AI within the TOPdesk platform. I will dig into the experiments that we have conducted, the capabilities that we have developed, what we have learned along the way, and give you a glimpse into the potential AI-based functionality that you can expect to see in the TOPdesk platform going forward.
AI Progress to date
AI is a pretty broad term and, confusingly, it is often used interchangeably with automation. This confusion is understandable. On a technical level, AI and automation have always been considered two separate areas. However, on a functional level, they both ultimately contribute to very similar goals (increased efficiency, productivity, data-centricity, etc.).
In fact, the boundaries between the two are rapidly blurring—meaning they now fall into one overarching category, ‘hyperautomation’, which we will explore later on in this post.
For example, AI chatbots use automation to carry out simple tasks like resetting a password. In the same way, there is automation baked into TOPdesk’s capabilities, though you may not be aware of the instances where we actually leverage AI itself. But that is not to say that they do not exist.
Take our Smart Categorization feature, for instance. This functionality suggests the three most likely categories for a call, making it much easier for operators to find the right category. These suggestions are made based on the combination of request and brief description and greatly reduce instances of miscategorizations.
- It can also be combined with an automation feature called ‘category-based routing’ which assigns a call to an operator group based on call category—thereby achieving almost-automatic routing.
- The best part is, this AI is updated nightly. It’s always improving.
Then there’s our Call Affiliation feature, which groups incoming calls to similar ones that your agents have previously dealt with (including calls handled by other agents). By doing so, agents can easily detect major calls and problems as early as possible, identify if solutions that solved previous—and related—calls would work in this instance, and easily spot duplicate recurring calls.
‘Failed’ experiments – but promising progress
As proud as we are of our AI-powered Smart Categorization and Call Affiliation features, there have been a few experiments we tried that did not end up succeeding. While these failures were frustrating at the time, the research that we conducted has played a key role in furthering our AI expertise and knowledge of what is (and is not) possible.
1. Risk Assesment
For example, we tried to develop a Risk Assessment feature that would predict whether a call falls within the existing service-level agreement (SLA). However, there are so many variables that could potentially impact how long an incident would take to resolve – so making clear-cut predictions was not only extremely difficult, but they might also turn out to be completely inaccurate. That does not mean that we have totally given up on this project – but we are going to pause its development for the time being.
2. Knowledge items
Likewise, we have also tried to build out real-time suggestions of Knowledge Items while people are creating an incident. The goal was to provide agents with on-the-fly recommendations about how to solve an issue when they are creating an incident. However, despite making promising progress, we realized that it would take significant time, energy, and resources to finish this project – so it has been put on hold for now.
3. Caller sentiment
We tried another experiment where we used AI to indicate caller sentiment, hoping this would aid prioritisation: the more stressed the caller, the greater the urgency. Multiple third-parties use this functionality, but we found that it’s not so easily translated into service management. Many inbound calls relate to issues like missing software or broken assets. Unsurprisingly, the AI considers such calls to include ‘negative sentiment’—even though the caller is actually being friendly or neutral. We found potential solutions, but they would have rendered the implementation very difficult.
To be honest, however, it feels a little wrong to classify all these experiments as ‘failures’.
While they have not resulted in any changes to the TOPdesk platform itself, we fully intend on picking back up where we left off and finalizing these projects when we have the necessary time and resources. Plus, we also learned a ton about technology and functional applications of AI.
The ultimate goal is providing users with proactive, context-based suggestions that will ultimately make their lives easier going forward.
What we have learned
Over the years, we have learned that it is not overly useful to focus on AI specifically – instead, we are seeking to develop our hyperautomation (as Gartner puts it) capabilities. This means that we want to build all-round solutions that leverage the best of AI, machine-learning (ML), and automation to deliver maximum impact and value to our customers.
These technologies are all interlinked and form part of the same overarching mission: to make software smarter, more context-aware, more personalized, more proactive, and more efficient.
We have therefore developed a number of features with this general goal in mind. For instance, our Knowledge Item Suggestion widget provides suggestions as to which Knowledge Item might be able to help you solve your particular problem.
Then there is our category-based Automatic Call Assignment feature that automatically assigns incidents to the right operator group based on how it has been categorized. Not only does this help speed up the process, but it also eliminates unwelcome mistakes where incidents are sent to the incorrect operator group. But we have a confession to make: this first version isn’t very smart:
- It essentially assigns the call to the next available operator in that chosen group, without taking into account other factors including priority and urgency.
The end goal is to make this feature so smart that it finds the best personal match of caller and operator based on estimated call duration, operator day planning, operators’ personal skills, and so on. We’re not quite there yet—but we have a good platform from which to build these capabilities out by using an iterative ‘smartification’ approach.
Both of these solutions align with our ultimate goal of providing users with proactive, context-based suggestions that will ultimately make their lives easier going forward. In other words, they give the same benefits of AI-based solutions – except that they are not technically AI itself.
Likewise, Action Sequences leverage the power of automation to help you create automated end-to-end workflows within both the TOPdesk platform itself as well as with other apps within our Marketplace ecosystem.
So, what is coming in the future?
As stated above, we do not want to think of AI as a goal to pursue in and of itself.
We see smart software as the foundation of the TOPdesk platform in the future and aim to integrate it into every aspect of the platform. However, this encompasses all elements of hyperautomation, rather than purely AI alone.
We cannot currently provide precise information for the features we are developing and when they might be released. However, rest easy in the knowledge that we are doing everything we can do to make the TOPdesk platform smarter, more proactive, and more effective for our users.
Stay tuned for the next blog post in this series where we will examine how we’re leveraging AI to improve how TOPdesk interacts with other apps in our Marketplace ecosystem.
Inspire others, share this blog